Access To High Quality Data Hinders AI Adoption
Artificial Intelligence (AI) has become an indispensable tool for communications service providers (CSPs) with its promises to revolutionize network and service operations, leading to unprecedented levels of automation. However, this transformation has its challenges. CSPs face many obstacles in their quest to deploy AI and effectively harness its full potential.
One of the most pressing challenges CSPs face in adopting AI is the accessibility of high-quality data. Quality data is the lifeblood of AI systems, driving accurate decision-making and predictive capabilities. Unfortunately, many CSPs are hindered by legacy systems characterized by proprietary interfaces that make accessing the data necessary for robust AI integration arduous.
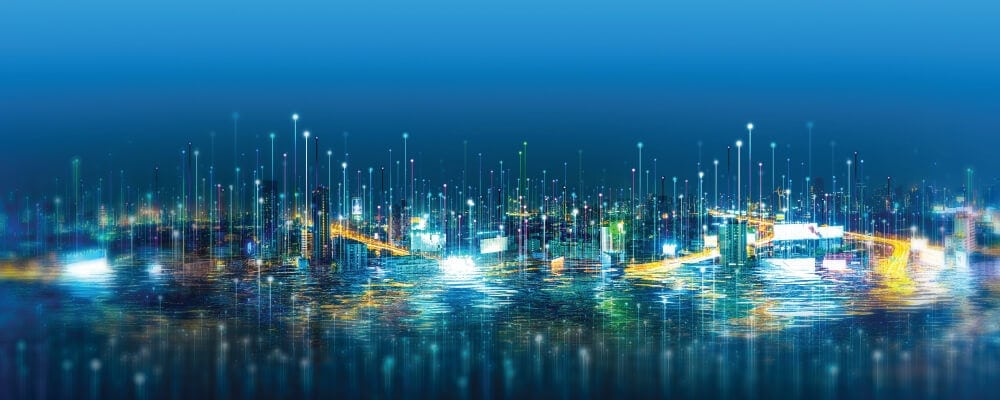
To understand these challenges better, Nokia partnered with Analysys Mason to conduct a comprehensive study on AI adoption within the CSP industry. This research surveyed 84 CSPs worldwide, shedding light on the current state of AI implementation and the hurdles these organizations face.
The findings of this research paint a vivid picture of the CSP landscape:
Limited Progress
Only 6% of CSPs surveyed believe they have reached the most advanced level of automation, often called “zero-touch automation.” This level relies heavily on AI and machine learning algorithms to manage and enhance network operations.
AI Implementation Underway
Despite the challenges, there is reason for optimism. The study reveals 87% of CSPs have initiated AI implementation in their network operations. This takes the form of proof of concepts or full production deployments. Impressively, 57% have already deployed telecommunications AI use cases into production.
The Promise of AI
CSPs foresee AI as a catalyst for improving network service quality, driving top-line growth, enhancing customer experiences, and achieving energy optimization, aligning with sustainability goals.
Data Access Is A Primary Challenge for CSPs When Adopting AI
Despite the promise of AI and the efforts made by CSPs, several challenges persist. As highlighted by the research, CSPs’ limited access to high-quality data is one of the biggest obstacles. Data remains siloed within current infrastructures and processes, making it challenging to develop AI-driven use cases. Data pipelines often fail to provide timely, high-quality data, exacerbating the problem.
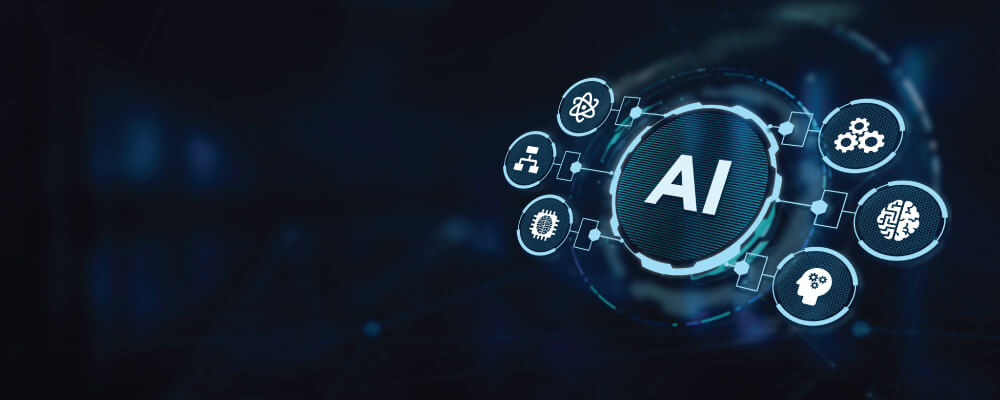
One challenge in the data collection stage is the proprietary interfaces of CSPs’ network infrastructure and systems that hinder the smooth flow of data during the collection process, particularly for Tier-1 CSPs operating extensive network infrastructure. Nearly 50% of Tier-1 CSPs surveyed in the research ranked data collection as the most challenging telecommunications AI use case development cycle stage.
These data-related challenges not only impede the integration of AI into the network but also affect CSPs’ ability to retain AI talent. Data scientists spend a significant portion of their time accessing and preparing data, leaving less time for deriving insights and contributing to the organization’s AI initiatives. This can lead to demotivation and increased attrition among data science professionals.
CSPs Require A Clear Blueprint For Deploying AI
Adopting a blueprint approach to implementing telecommunications AI use cases brings several advantages. By initially deploying use cases focused on optimizing network infrastructure operations, CSPs position themselves to better support customer needs, especially those in the enterprise market. Implementing domain- or infrastructure-specific use cases also lays the foundation for more advanced, cross-domain use cases like end-to-end service orchestration.
Additionally, CSPs gain valuable knowledge and expertise from deploying short- to mid-term use cases, which can be leveraged to drive long-term use cases. This iterative approach not only enhances operational efficiency but also helps CSPs realize the full potential of AI in diverse aspects of their operations.
Exploring Cross-Domain Use Cases
One of the critical aspects that CSPs should consider in their AI journey is the exploration of cross-domain use cases. These use cases leverage insights from deploying short- to mid-term use cases to support long-term strategic objectives.
Short-term use cases often focus on optimizing network infrastructure operations. These use cases can include automated incident detection, alarm reduction, health checks of network infrastructure, energy optimization, and intelligent capital expenditure allocation. What sets them apart is that they can be relatively easy to deploy, as the data required for training AI models can be sourced from internal systems within the same network organization. Additionally, access to data is typically granted by internal CSP network stakeholders.
Mid-term use cases introduce more complexity and address broader network concerns, requiring data from multiple network domains (access, core, transport) and non-network related teams. For example, end-to-end service orchestration would require AI/ML models capable of detecting issues across various network domains and initiating remedial actions through the orchestrator.
Long-term use cases, driven by the need to capture new revenue opportunities, are often the most complex. They necessitate access to third-party data sets and external industry expertise. Examples include:
- AI for video analytics.
- IoT device analytics (such as smart manufacturing and autonomous driving).
- Other data-driven services beyond traditional connectivity offerings.
Implementing these use cases requires substantial time and effort due to the complexities of data access, pre-processing, and model development.
Vendor Partnerships Should Focus On Expertise In Telecoms, AI, and Software
As CSPs embark on their AI journey, forming strategic vendor partnerships becomes imperative. These partnerships can significantly accelerate AI projects and provide access to the expertise required for successful AI development and deployment. However, selecting the right vendor partners is essential, and specific criteria must be considered.
Combined Telecommunications
and AI Expertise
Vendors should possess deep knowledge in both telecommunications and AI. This combination of expertise allows them to understand CSPs’ networks, operations, and pain points, enabling them to design, deploy, and manage AI solutions effectively.
Managed Services
Capabilities
Vendors should offer managed services capabilities to support CSPs’ AI initiatives. This includes data and model management expertise, ensuring that the AI solutions remain robust and reliable.
SaaS Delivery Model
CSPs find Software-as-a-Service (SaaS) delivery models attractive due to their ease of use, scalability, and agility. Vendors offering AI solutions as SaaS should align with CSPs’ stringent security requirements, particularly regarding data storage and management.
Leveraging Cloud Platforms
Cloud-based AI and data platforms are gaining popularity among CSPs. Vendors can leverage these platforms to deliver AI solutions, enabling CSPs to access innovative data and AI services from hyperscalers and other cloud solution providers.
Platform-Based Approach
Vendors should adopt a platform-based approach for delivering AI use cases. This approach allows for the reuse of platform capabilities, accelerating AI development and enabling the extension of assets to support new use cases. It also allows CSPs to develop use cases tailored to their specific needs.
Nokia’s AIOps Leads The Way In AI Adoption For Telecommunications
AI and automation, while not interdependent, create a powerful synergy when used in tandem, leading to an elevated level of optimization in network and service operations. AI Operations (AIOps) empowers organizations to harness the entirety of network events – past, present, and future – and proactively address issues before they manifest. This strategic integration also yields significant benefits, such as substantial customer experience enhancement. AIOps applications extend to areas such as network planning, cost-effective roaming management, and improved network energy efficiency.
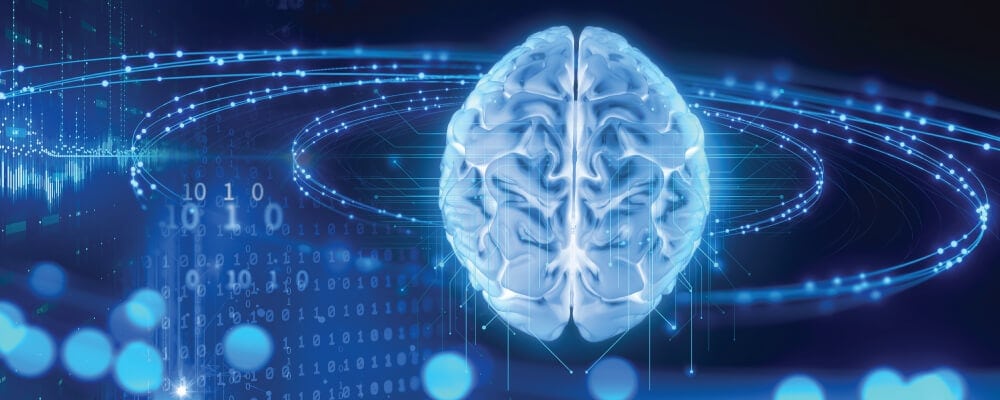
Within the telecommunications industry, Nokia stands as a recognized authority in AIOps. Leveraging a well-established blend of networking proficiency and software acumen, Nokia’s AI Operations portfolio champions automation and intelligence across service domains, delivering direct benefits to end customers. With a rich history of expertise in OSS/BSS and substantial investment in AI innovation at Nokia Bell Labs, they are at the forefront of applying AI in the telecommunications sector. Their unique position allows the organization to guide CSPs in choosing the most appropriate AIOps methodologies, determining the optimal timing for implementation, and understanding the underlying reasons for these choices – all in the present context.
About Infinity Technology Solutions
Infinity Technology Solutions specializes in broadband and critical communications infrastructure development. We help our channel partners create private wireless networks 4G/5G, microwave backhaul systems, IP/MPLS, and optical networking technology.
For more information, give us a call or fill out the contact form below.