AI and ML Play Transformative Roles
Broadband access has become a fundamental human right, crucial for our digital economy. The rapid growth of subscribers, data, and speed is changing traditional broadband paradigms. Emerging technologies like 5G, smart homes, and Industry 4.0 require networks that can handle symmetrical traffic, low latency, and constant connectivity.
Traditional network skills are no longer enough to manage this complexity. Automation is essential to streamline operations and reduce errors. Network elements are shifting to software and cloud-based solutions, creating a need for an IT-centric approach. This approach improves efficiency and agility. Cost reduction pressure drives hardware performance innovation, including less resource-intensive algorithms.
Artificial Intelligence (AI) and Machine Learning (ML) play transformative roles in these areas, complementing or even surpassing existing algorithms. They enhance decision-making, adapt to changes, and respond quickly to threats.
Incremental Steps in Automation
The telecom industry has a long history of technological advancements, evolving from manual operations to highly automated systems. The journey towards automation in the broadband industry is currently in its midpoint. It’s essential for maintaining network services.
This evolution began in the early 20th century with the introduction of automated telephone exchanges, freeing telephone operators from the task of manually connecting calls using cord pairs on switchboards. Since then, the march of automation has been relentless, reshaping communications in profound ways. While the industry has achieved unprecedented levels of efficiency, the expertise and skills of human professionals remain indispensable in ensuring the seamless operation of networks.
The initial phase relied on inflexible, manual processes. Transitioning to the second phase involves embracing open APIs, where Nokia is actively involved in fostering open initiatives and standardization efforts. Open APIs simplify network expansion with various technologies and vendors, leading to resource savings and enabling more innovation and value delivery to customers.
Nokia plays a significant role in fostering open initiatives and collaborating with standardization bodies to establish shared data models that the entire industry can utilize. The implementation of open APIs simplifies the process of expanding networks with various technologies and vendor solutions. Standardization and interoperability serve as essential cornerstones, offering substantial resource savings for both network operators and vendors. This efficiency arises from the reduction of prolonged integration and testing cycles, enabling them to redirect their efforts towards innovation and delivering enhanced value to their customers.
The Final Frontier: Automated Networks
The telecom industry is moving into a new phase of automation. In the current model-driven phase, programming is crucial. The next phase introduces intent-based automation, where operators define service policies, and the network self-adjusts and fixes issues automatically.
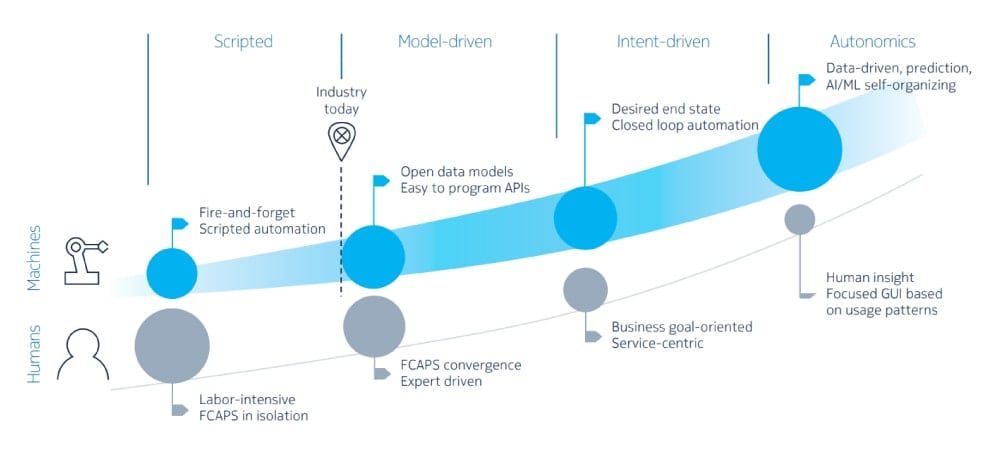
The final phase envisions a self-aware, data-driven network using AI and ML to automate operations, predict and prevent issues, and offer insights for anomaly detection, action recommendations, and capacity planning. This approach reduces the need for manual scrutiny and is a key part of Nokia’s evolution.
Innovate and Develop
Nokia’s corporate culture is deeply rooted in R&D automation. This approach ensures quality and transparency in agile technology development, benefiting operators with improved products, faster time-to-market, and reduced flaws. It relies on contemporary requirement-gathering, intuitive software development, and automated workflows from feature inception to delivery. A robust solution regression capability identifies issues early through daily tests bolstered by DevOps practices and a cutting-edge CI/CD pipeline. Quality metrics like code churn and coverage guide daily auto-regression and testing, maintaining product excellence.
Marketing and Sales
In regard to marketing and sales, two distinct groups of tools emerge. The first group is operationally focused, serving marketing, sales, and customer relationship management functions. These tools enable swift responses with personalized communication and assist in order processing, lead nurturing, managing the sales funnel, and understanding consumer behavior. They also facilitate the identification of network areas with spare capacity, automatically notifying marketing and sales teams to strategize and execute campaigns for selling additional services.
The second group of tools is strategically oriented, aiding in the modeling of new customer services and recognizing market opportunities for emerging technologies such as 5G, fiber-to-the-home, and software-defined networking. These tools amalgamate technological, market, and business model knowledge, assisting operators in making strategic decisions through automated return on investment (ROI) and total cost of ownership (TCO) models. Leveraging Nokia Bell Labs’ patented algorithms, these tools enable the accurate modeling of tailored strategies for launching new services and evolving broadband networks.
Plan and Verify
Strategic investment in planning, design, integration, and validation processes is crucial for minimizing risks, costs, and delays during network deployment and operation. These processes involve advanced design algorithms and user-friendly interfaces for network planning, dimensioning, assurance metrics, capacity management, and resource planning. For instance, intelligent tools automate the design of physical fiber infrastructure, optimizing factors like homes passed, subscriber take rates, costs, and more. Additionally, design tools assist in selecting the best migration path for PSTN migrations by gathering data from voice switches. This phase also includes iterative integration and verification activities to ensure the network’s performance and operational robustness before deployment.
Activate and Perform
Nokia is a leader in bringing fixed services to subscribers, catering to both established and emerging fixed-access technologies. Nokia assists carriers in speeding up broadband service delivery through easy device setup, consistent configuration management, and automated service provisioning. For instance, they guide users and technicians in installing wireless CPEs optimally and ensure service quality through dynamic cell assignment, coverage/capacity algorithms, and post-installation diagnostics.
They simplify FTTH activation, transforming it into an automated process and eliminating the need for appointments or on-site technician visits. Their solutions, like Nokia WiFi Beacons and gateways, use smart automation and mesh technology to optimize Wi-Fi performance, enhancing the end-user experience. Additionally, Nokia enables network optimization through cloud controllers, improving metrics for entire areas.
Nokia optimizes network rollout with end-to-end delivery automation, ensuring efficient cooperation among stakeholders, reducing rollout costs by up to 30%, and minimizing errors. The company has a history of successful broadband network transformations and offers automation in migration processes, including PSTN, ATM, IP, GPON to XGS-PON, and SDN migrations.
Their Software-defined Access Networks introduce zero-touch operations, making nodes ready for service without manual configuration, resulting in increased flexibility and cost savings. They also introduce intent-based networking (IBN) to simplify network integration and automation. Operators define desired outcomes, and the network self-configures to support them, enhancing network robustness and adaptability.
Maintain and Support
Nokia is a leader in delivering fixed services to subscribers, covering both established and emerging fixed-access technologies. They help carriers accelerate broadband service provision with user-friendly device setup, consistent configuration management, and automated service provisioning. For instance, Nokia guides users and technicians in installing wireless CPEs for optimal service quality using dynamic cell assignment, coverage/capacity algorithms, and post-installation diagnostics.
Nokia streamlined FTTH activation, transforming it into an automated process and eliminating the need for appointments or on-site technician visits. Solutions like Nokia WiFi Beacons and gateways leverage smart automation and mesh technology to optimize Wi-Fi performance, enhancing the end-user experience. Additionally, Nokia enables network optimization through cloud controllers, improving metrics for entire areas.
Nokia optimizes network rollout with end-to-end delivery automation, fostering efficient collaboration among stakeholders, reducing rollout costs by up to 30%, and minimizing errors. With a successful track record in broadband network transformations, Nokia offers automation in migration processes, including PSTN, ATM, IP, GPON to XGS-PON, and SDN migrations.
Their Software-defined Access Networks introduce zero-touch operations, making nodes ready for service without manual configuration, resulting in increased flexibility and cost savings. Nokia also introduced intent-based networking (IBN) to simplify network integration and automation. Operators define desired outcomes, and the network self-configures to support them, enhancing network robustness and adaptability.
Principles of Artificial Intelligence
The incorporation of AI at the core of applications reduces the dependence on human-crafted algorithms. AI systems have the capacity to recognize patterns in data and acquire knowledge from it without the need for explicit programming. In suitable use cases, AI can autonomously construct models that determine the most effective responses to a wide range of inputs, circumventing the necessity for conventional software development.
The extent to which this technology can advance is still a matter of exploration. The potential of augmenting human intelligence with AI holds the promise of ushering in a new era where civilization can thrive like never before, potentially initiating the fourth industrial revolution.
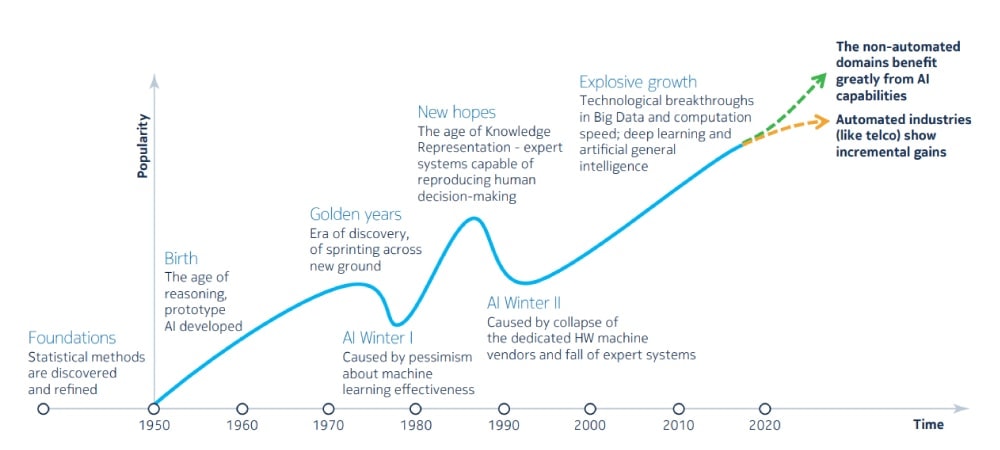
In the context of service provider networks, these networks have already undergone significant automation phases and operate at a high level of efficiency. While researchers may be grappling with initial disillusionment, recent developments in AI and ML in the telecom industry have shown encouraging results. It is expected that these advancements will continue to enhance productivity for a widening array of tasks, particularly because AI’s efficacy is rooted in its ability to learn from data. In today’s world, there is an abundance of data available to train AI algorithms, thanks to the proliferation of the Internet, cloud-based applications, and the widespread adoption of the Internet of Things (IoT). This surge in data volume and sources has created a fertile ground for AI and ML to thrive in the telecom sector.
AI is a broad term within the field of computer science that refers to any human-made device or system capable of perceiving its environment, making decisions, and taking actions aimed at maximizing its likelihood of achieving a specific goal. There are various approaches to building AI systems, one of which involves the explicit programming of clever rules. This approach, often referred to as “good old-fashioned AI,” was the dominant trend in the past. However, it comes with the drawback that whenever a new problem arises, programmers must manually rewrite the rules, which can be impractical.
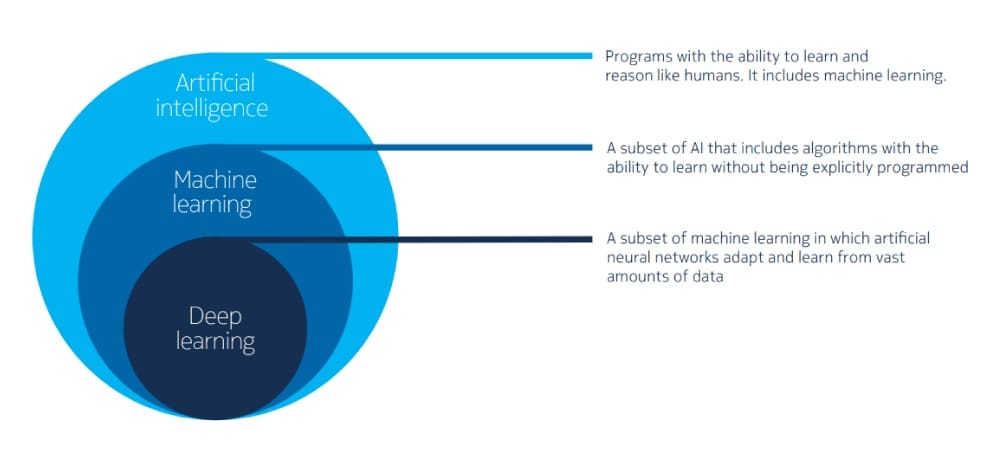
Machine Learning is a subcategory of AI that offers computational systems the ability to learn tasks without explicit programming. ML systems excel at recognizing patterns in data and acquiring valuable insights from that data. Many of the recent breakthroughs in AI have been a result of successful ML implementations. Deep Learning, a specialized class of machine learning, is built on deep neural networks capable of modeling complex relationships between input features and target outputs.
AI is still in its early stages in the telecommunications industry. While a number of organizations have started to explore AI, recent research indicates that almost half of all service providers have not yet implemented any AI initiatives. Among those who have initiated AI projects, the focus tends to be on enhancing existing business processes through actions such as the recognition of patterns, the detection of anomalies or irregularities, and the subsequent actions taken to address the identified problems. This application of AI is instrumental in enhancing the efficiency and reliability of network operations and services.
Anomaly Detection
The fundamental concept behind real-time anomaly detection is to compare live data being collected with a reference or expected range that is known to represent a normal, healthy condition. Various levels of anomaly detection algorithms differ in how they determine this expected range. In its simplest form, this range is manually defined by a domain expert, with these key performance indicator (KPI) values set once and updated infrequently through manual intervention.
To scale and improve this approach, automatic fingerprinting can be employed to set the expected range automatically, particularly after the deployment of a new service. However, this mechanism has limitations when it comes to KPIs that exhibit a cyclical or seasonal pattern due to factors like temperature or network traffic variations. These patterns can complicate the detection of true anomalies.
This is where AI and ML come into play. AI/ML leverages well-established techniques, including rolling mean, standard deviation, and auto-regressive algorithms (such as ARIMA), to differentiate seasonal and cyclical patterns from the underlying trend and background noise. This allows for a more accurate prediction of the expected range and significantly improves the speed and accuracy of anomaly detection.
Additionally, advanced deep learning techniques, like convolutional or recurrent neural networks, which have proven effective in time series prediction, can be utilized for even more precise anomaly detection. Real-time anomaly detection is useful in various scenarios, including identifying the degradation of optical signal power, traffic spikes in the network, abnormal or unfair user data consumption, and monitoring metrics like board temperature, CPU, and RAM usage.
Closed-loop Automation and AI/ML
Detecting anomalies is just the first step – it’s just as important to identify the root cause of these anomalies and take appropriate remedial action, especially if they lead to service degradation. Various levels of complexity exist for automating the remediation process. In simpler cases, where the remedy action can be directly derived from the detected anomaly, a straightforward rule engine can be employed. These rule engines work on a set of “if-this-then-that” type rules and multiple rules can be combined to tackle slightly more complex problems. However, these rules need to be manually configured and maintained by qualified personnel.
For more complex problems, ML can provide valuable assistance. Reinforcement learning, for example, is a technique that can automatically identify the most effective configuration changes to apply to a system (or network) in order to maximize a specific objective, such as network stability, performance, or equitable resource sharing. This approach is particularly beneficial in complex scenarios where the number of potential configuration changes is vast and a dynamic, adaptive response is required.
AI/ML For Fixed Application Networks
Nokia envisions the widespread use of ML across all levels of the fixed network to enable quicker, more informed, and more predictable decision-making. This approach involves focused problem-solving and a deep understanding of the domain, coupled with modeling and simulations of system behavior.
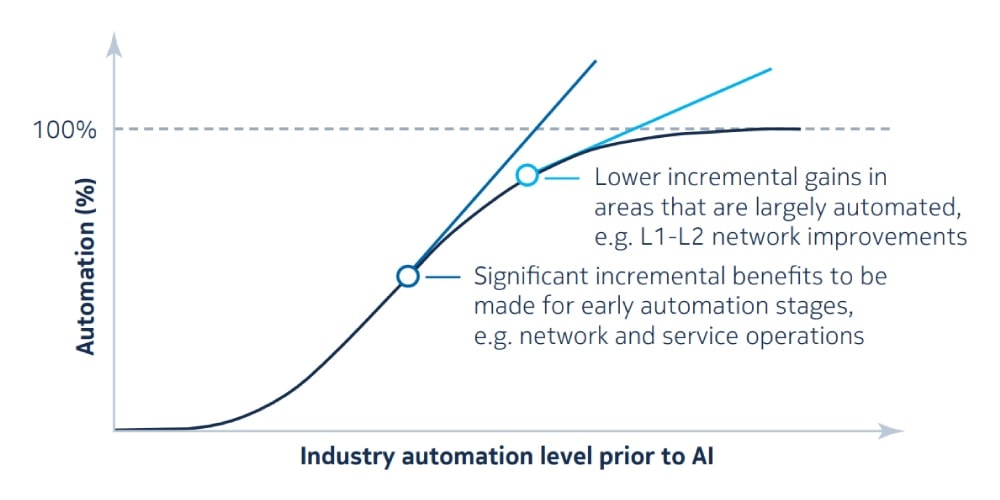
In the context of Layer-1 and Layer-2 optimizations, the benefits of AI/ML are expected to be incremental rather than disruptive. This is because these layers are already highly optimized, and the goal is typically to achieve optimization targets in the 10% to 20% range. Conversely, areas involving human resources – such as operations and development – are anticipated to yield higher gains, typically in the range of 30% to 40%.
Nokia’s comprehensive portfolio spans the entire spectrum of AI solutions, encompassing AI-as-a-Service, platform components, hardware products, network management, and dedicated software components equipped with AI and ML capabilities.
Innovate and Develop
Nokia places a strong emphasis on software quality prediction and employs data-driven engineering techniques to tackle quality control challenges in software development. ML-based analytics play an important role in predicting software quality using a wide range of parameters, including code lines, test lines, commits, reviews, story metrics, and more.
Here are some key aspects where AI and ML are leveraged to enhance software development and quality control:
Software Development
Defect Identification: ML is used to screen code and automate the review process, highlighting sections of code that require human attention. This not only helps in identifying defects or design issues but also streamlines the code review process.
Recommender Systems: Recommender systems assist developers by providing automated, intelligent suggestions for code items that are relevant to their current tasks. This accelerates decision-making and code integration, ultimately leading to faster and higher-quality development.
Test Suite Automation
Regression Testing: ML technology is employed to automate the selection of regression test cases based on code changes. It can also predict potential solutions for failed test cases. By proactively filtering and mapping source code and intelligently selecting test cases, this approach significantly reduces the overall time required for regression testing.
These AI and ML-driven approaches not only improve software quality but also enhance the efficiency and effectiveness of the software development process, ultimately leading to higher-quality products and faster development cycles.
Telemetry Design for Serviceability
Designing telemetry for serviceability is a key aspect of measuring system performance. While higher-resolution data collection provides more detailed information, it also introduces more overhead costs. It’s essential to strike a balance when designing data telemetry to meet the requirements of predictive care and closed-loop automation without adversely affecting the performance of network elements.
Automated Log Analysis
One of the challenges in log analysis is that similar events in logging data are not always easy to detect manually. ML learns to automate the process of log parsing and analysis, drawing from past experiences with captured events, recognized symptoms, and classifiers developed during lab testing. With this automated log analysis, ML systems can identify the most likely root cause of an issue, specify the affected software modules, and automatically route the problem to the appropriate team for resolution. This streamlines the serviceability process, making it more efficient and effective in identifying and addressing network element issues.
Marketing and Sales
Personalized Service Recommendations
ML-driven personalized service recommendations empower marketing and sales teams to cultivate leads and precisely target clients effectively. Many consumers may not be fully informed about the availability of advanced service options or may lack clarity on what to anticipate. AI/ML technologies enable the identification of upsell opportunities and the tailored recommendation of the most suitable services for each individual, taking into account their unique traffic patterns.
Plan and Verify
Radio Resource Management
Radio resource management encompasses the intricate control of numerous parameters, including transmit power, user allocation, data rates, modulation schemes, error coding, and more. The primary goal is to optimize the utilization of the finite radio-frequency spectrum to be as efficient as possible. Leveraging ML techniques, such as intelligent schedulers and interference coordination, can significantly enhance throughput while reducing latency.
Optical Channel Equalization
The performance of fiber-optic communication systems, particularly when operating at Gigabit speeds, is affected by channel interference and dispersion. AI-driven digital signal processing plays a role in expediting fiber channel equalization by preloading equalizer coefficients. It also enhances receiver sensitivity through AI-based channel inversion and non-linearity cancellation, not only improving system performance but also offering cost savings by enabling the use of higher power classes with the same optical components.
Capacity Planning
Relying solely on historical data is insufficient for accurately predicting traffic congestion in advance. ML can revolutionize capacity planning by proactively monitoring resource and network utilization, issuing notifications before capacity depletion, or identifying capacity concerns during network planning. Leveraging ML techniques, networks can learn from telemetry data to discern actual traffic patterns influenced by subscriber behavior. This, in turn, facilitates the prediction of Service Level Agreement (SLA) conformance, such as the probability of success in speed tests.
Plug and Play Node Configuration
Machine learning can acquire configuration best practices from previous deployments and suggest the appropriate settings during the initial connection process. Traditionally, configuring DSLAM or OLT equipment demands expert engineering skills and adhering to predefined rules, making the installation process challenging. Operators, however, seek solutions where DSL or PON connections can function seamlessly “out of the box,” thereby expediting the time-to-market for their services.
Activate and Perform
Physical Layer Optimization
Determining the optimal parameter configuration for digital beamforming in massive MIMO systems is a complex endeavor. The ideal setup varies based on factors like the physical environment and user distributions. In such scenarios, the application of ML can significantly enhance user throughput without introducing excessive implementation complexity.
In the fixed access PHY layer, there exists a high degree of flexibility regarding parameters like time slot (cDTA), spectrum allocation, and power allocation. The optimal configuration is contingent on variables such as traffic volume and user behavior patterns, encompassing high-speed internet (HSI), video streaming, and remote work activities. Manual parameter tuning necessitates extensive expertise because the ideal settings can differ for each network node.
Additionally, indoor positioning achieved through Wi-Fi sensing and neural networks can deliver remarkably precise data concerning position, presence, motion detection, device tracking, home security, building automation, and healthcare systems. This technology holds the potential to revolutionize a wide range of applications and services.
Smart SLA Management
ML can play a pivotal role in the verification of service compliance with Service Level Agreements (SLAs) and the prediction of near-future capacity trends. Ensuring SLA adherence, which encompasses throughput, error rates, and latency, is imperative for operators. They not only aim to confirm that existing SLAs are met but also to guarantee future compliance as more users, services, or virtual network operators become active on the same network.
Traditionally, operators have relied on data-capping techniques to manage network resource allocation. However, these methods may not guarantee fair bandwidth sharing and optimal network utilization. By implementing AI/ML-driven closed-loop automation, it becomes feasible to enhance control mechanisms, optimize network performance, and ensure equitable resource allocation.
Maintain and Support
Predictive Care
AI/ML technologies play a crucial role in the proactive monitoring of network trends and the early identification of problematic conditions. This results in the swift and highly accurate detection of the root causes of issues. As a result, outage durations can be reduced by 34%, and the resolution of problems can be accelerated by 40%.
Additionally, the integration of RPA (Robot Process Automation) and intelligent ticket analysis into the support process automates tasks that are repetitive and rely on structured data. This streamlines operator workflows and enhances efficiency.
Digital assistants and Natural Language Processing (NLP) technologies are also widely employed, particularly in the form of conversational agents and chatbot services. These tools assist in rapidly addressing user inquiries and finding solutions to various problems, enhancing the overall customer support experience.
Outside Plant Analysis
Copper channel fault diagnosis is crucial for identifying the locations of shorts and open circuits, detecting bridged taps or load coils, and pinpointing disturbances in channel frequency response. In the event of DSL service interruption, it is vital for operators to determine the fault’s precise location, whether it resides at the central office or the customer’s premises, and to identify the nature of the fault, such as a bad contact or a malfunctioning Customer Premises Equipment (CPE). Deep learning enhances the capabilities, accuracy, and comprehensiveness of contemporary line diagnostic tools like Hlog and SELT, resulting in more effective fault diagnosis and resolution.
Uncontrolled crosstalk and noise disturbances can significantly impact vectoring performance and DSL stability. It is possible to detect both known signatures and abnormal, previously unidentified patterns. AI can be harnessed to correlate error behavior across the lines, eliminating poorly performing lines or notch bands to enhance overall system performance.
Additionally, issues stemming from fiber bends and optical connector problems can lead to transmission errors that are challenging to isolate. AI, in conjunction with Optical Time-Domain Reflectometer (OTDR) and Received Signal Strength Indicator (RSSI) parameters, can swiftly identify and locate these issues, expediting the repair process and bolstering the efficiency of field service teams.
Predictive Hardware Maintenance
The application of ML to online diagnostics empowers the detection of ports displaying non-linear, unstable behavior, often induced by factors like lightning strikes. ML algorithms are capable of monitoring crosstalk, error rates, and retransmission counters, swiftly identifying and flagging such unstable ports. Additionally, AI plays a crucial role in preventing service interruptions by detecting when a board is nearing a reset, which it accomplishes by monitoring CPU and RAM usage and implementing proactive measures to ensure service continuity.
Additionally, AI demonstrates its value by detecting gradual degradation in optical transceiver performance, including sensitivity and transmitted power, often attributed to aging components. It can offer recommendations for replacing these components before transmission errors or service disruptions occur, optimizing network reliability and performance.
Incident Advisor Systems
Conventional network operations rely on rule-based systems for problem identification and resolution. However, the introduction of ML expands the range of network behaviors that can be analyzed, offering insights into correlations and causations without the need for pre-defined rules. Leveraging event noise reduction algorithms, efficient clustering techniques are employed to identify incidents. Additionally, through supervised classification, a knowledge base can be developed, enabling the recommendation of solutions and the implementation of well-vetted workflows for issue resolution. This not only enhances network management but also optimizes operational efficiency.
Quality-of-Experience Optimization
PHY-layer Service Level Agreements (SLAs) are typically specified in terms of throughput, error rates, and latency. However, customers assess the quality of service at the application layer, where factors like web responsiveness and video stream quality, including pixelization and buffering issues, are crucial. Establishing a straightforward relationship between PHY-layer SLAs and perceived service quality can be challenging.
AI plays a pivotal role in bridging this gap by predicting application quality based on PHY-layer parameters. This approach ensures an optimal subscriber experience by aligning network performance metrics with the user’s actual perception of service quality.
AI Cyber Security
The increasing volume and complexity of cyber threats present significant challenges. AI has demonstrated its potential to enhance threat detection and introduce valuable time-saving automation into security operations. By leveraging AI, security teams can improve their responses in the face of ambiguity, transition to a probabilistic model of security operations, and effectively identify malware infections within the communication network.
Data-Centric AI Strategies
The effectiveness of any AI application is closely tied to the quality of the data it relies on. In this context, data becomes the most critical component of the entire AI system. Recognizing this, Nokia Bell Labs has developed a Future X network architecture with a strong focus on data collection. This approach ensures that the capture, transmission, storage, and processing of vast datasets are integral to the hardware requirements and fundamental algorithms of Nokia’s network solutions.
Key principles guiding this data-centric approach include:
- High-Precision: Data is collected in a complex yet appropriately structured format, encompassing configuration settings, logs, alarms, counters, and more.
- Centralization: The strategy avoids fragmented and incomplete views by aggregating inherently distributed data into a centralized system.
- Consistency: It emphasizes the importance of fast telemetry for real-time streaming as well as historical data collection.
- Standardization: Non-proprietary data sets are employed, allowing for direct comparisons and compatibility with ML algorithms.
- Future-Proofing: High-quality data is made readily available in the cloud, ensuring accessibility when needed.
- Security and Privacy: Stringent adherence to Advanced Information Management (AIM) and General Data Protection Regulation (GDPR) requirements.
- Platform Openness: Operators are provided with the flexibility to introduce their own models and algorithms for processing network data.
It’s essential to recognize the substantial amount of data typically required for implementing an ML system, often involving millions of data points. Additionally, in some instances, extensive data may not be necessary to maintain an adequate service level, but the system’s performance can continually improve by collecting more data during service usage.
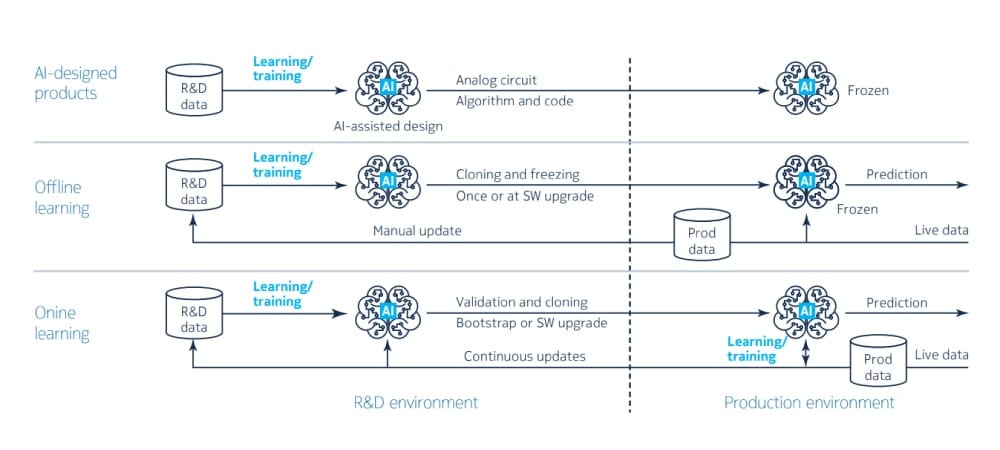
AI-Designed Products/Components
Over the past several decades, research and development engineers have relied heavily on numerical simulators for the design of analog circuits. These design tools excel in optimizing individual circuit components. However, as the complexity of circuits grows, the task of identifying the optimal values for each component becomes progressively more challenging.
To address this challenge, AI and ML techniques – such as genetic algorithms or reinforcement learning – are emerging as valuable tools. These techniques offer the potential to provide superior results and are already applicable today, particularly in the design of filters or antennas. Additionally, this same concept can be extended to optimize the configuration parameters of algorithms embedded in network equipment software.
Offline Learning
Offline learning algorithms are trained outside of the production environment, typically in a two-phase process. In the initial phase, the model is trained during product development using an initial dataset. Later, the model’s performance can be enhanced by retraining it with new batches of data. Offline learning offers more control as the model’s output can be optimized step by step.
This approach is commonly used with supervised learning, which depends on labeled data. However, obtaining accurately labeled data is often a difficult task, requiring manual work or the expertise of data scientists. Additionally, models may need to be periodically rebuilt to maintain their accuracy over time.
An example of offline learning application is predictive maintenance algorithms that forecast equipment failures in network equipment and passive elements like cables and connectors.
Online Learning
Online learning is a commonly used technique in situations where training over a single dataset is impossible or undesirable. These algorithms update their parameters sequentially as new data becomes available directly from the production environment. Online learning is essential in cases where it’s necessary to dynamically adapt to changing data patterns or when all training data is not available simultaneously.
However, online learning comes with challenges, such as testability and vulnerability to deviations. Therefore, monitoring and validating the learning process are essential to ensure that predictions remain accurate over time.
Examples of online learning applications include vocal assistants that learn the voice characteristics of individual users and network nodes that learn the traffic habits of connected customers, enhancing their configurations accordingly.
The Role of Humans and Domain Expertise
AI has raised concerns about the displacement of human workers, but history has shown that automation doesn’t necessarily lead to job loss. Automation can both create and eliminate jobs, with the key factor being how it complements human work styles. Automation often leads to the redirection of human workers toward more productive tasks.
In the case of AI, early adopters have faced the challenge of reskilling their workforce for new, augmented roles and have sometimes struggled to find enough trained staff. Future management and orchestration systems will harness the strengths of both humans and computers. Automated solutions offer consistency and rapid problem-solving capabilities, making them essential for addressing issues affecting network performance promptly.
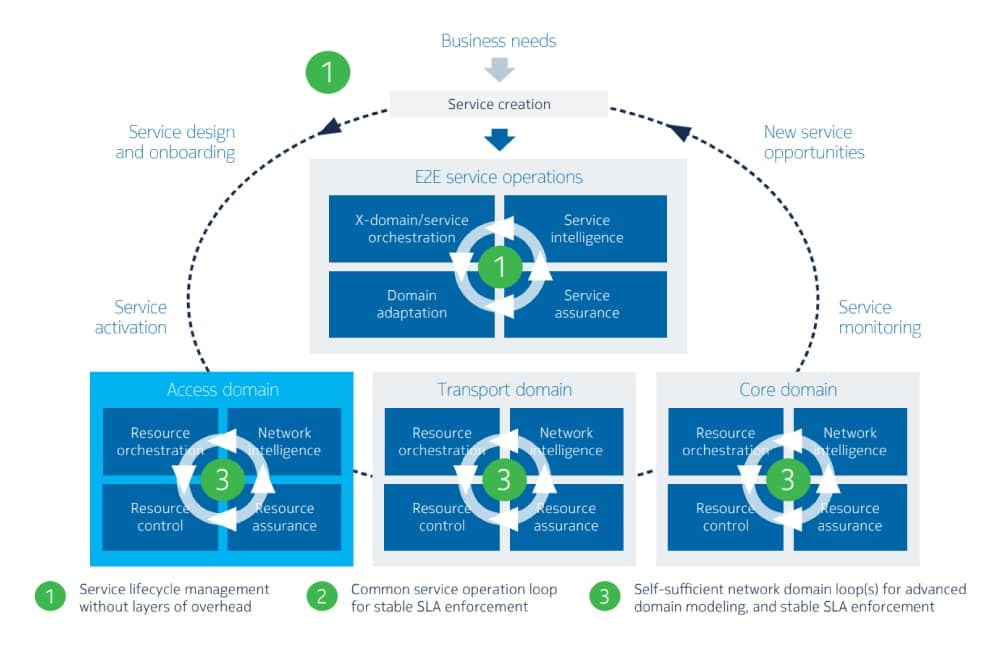
However, human operators possess unique strengths, such as their ability to make strategic decisions that might not seem immediately logical, like enhancing service quality in one area while implementing energy-saving measures in another. When network engineers are effectively assisted by AI-driven tools, it often leads to higher job satisfaction and opportunities for upskilling, as the synergy between humans and machines can be a powerful combination for achieving optimal results.
Within each control loop, the human operators play an important role since they have the autonomy to remain in control and can decide whether to select, postpone, ignore, or auto-execute the recommendations generated by data-driven analyses. This collaborative approach ensures a balance between human oversight and AI-driven insights, resulting in effective decision-making and network management.
Download a Copy of Nokia’s White Paper >>
About Infinity Technology Solutions
Infinity Technology Solutions specializes in broadband and critical communications infrastructure development. We help our channel partners design and deploy private wireless, microwave backhaul, IP/MPLS, and optical networking technologies.
For more information, give us a call or fill out the contact form below.